Are you confident that your Business Intelligence tool is as smart as it claims to be?
How often do you encounter discrepancies between the insights generated by your BI reporting tools and the actual outcomes? In the fast-evolving world of data analysis, having a Business Analytics tool that genuinely meets your needs is more crucial than ever. While hopes are high, reality often falls short.
While 97% of organizations are investing in big data and AI, only 29% of those surveyed believe they have derived actionable insights from their data. This gap is a critical disconnect between the capabilities of Business Analytics solutions and their practical applications.
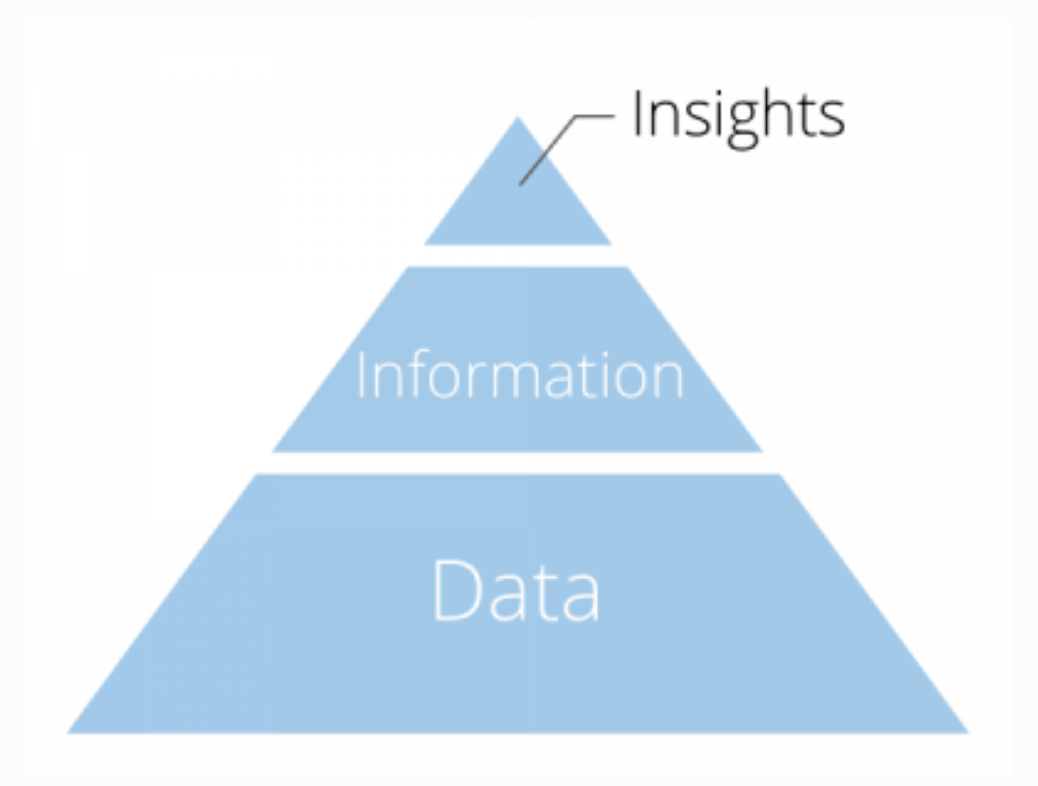
As we dive deeper, we'll explore the complexities and limitations of current Business Analytics tools. From overestimated automation capabilities to the nuances of data quality and context, we will uncover why these tools might not be as intelligent or as universally applicable as you might think.
Join us as we dissect the myths and realities of Business Intelligence tools, setting the stage for a more informed approach to leveraging these technologies in your business.
Key Limitations of Current Business Analytics Tools
Data Quality and Integrity
One of the most significant challenges faced by Business Intelligence tools is the quality of the data they process. BI reporting tools are only as good as the data fed into them. Poor data quality—be it incomplete data sets, incorrect entries, or outdated information—can lead to inaccurate outputs and misguided business decisions.
For instance, a common issue in BI implementations is the failure to maintain clean and updated data repositories. When Business Analytics tools process this compromised data, the insights generated can lead to flawed strategic decisions. This was the case for a retail chain that relied on outdated customer data, resulting in a marketing campaign that targeted the wrong demographic, wasting substantial resources and opportunities.
Lack of Contextual Understanding
While Business Analytics tools excel at processing large volumes of data, they often lack the ability to understand the context behind the data. This limitation is critical because data without context can mislead rather than guide.
For example, during an economic downturn, a sudden drop in sales might be interpreted by a Business Intelligence tool as a performance issue rather than a market-wide trend. Without the ability to contextualize data, companies might wrongly penalize certain departments or make poor strategic decisions. This lack of contextual understanding emphasizes the need for human oversight in interpreting what the analytics tools report.
Over-Reliance on Automation
Automation in Business Analytics solutions is intended to streamline processes, reduce labor costs, and improve efficiency. However, an over-reliance on automation can lead to significant issues, particularly when unexpected anomalies or new trends occur in the data.
A relevant case involved an automated BI system that failed to flag a gradual but significant decline in product quality, which was reflected in customer feedback and return rates. The system was programmed to monitor sales volumes and revenue but not customer satisfaction metrics directly. This oversight led to a continued decline in brand reputation and customer loyalty, which took months to identify and address.
The Misconception of 'One-Size-Fits-All' Business Analytics Solutions
Businesses frequently deploy the same BI reporting tools across various departments without considering the unique analytical needs and data processing requirements of each. For instance, the marketing department and the finance department have vastly different data analytics needs. Marketing might require deep insights into consumer behavior and campaign performance, involving lots of unstructured data and sentiment analysis, which are less critical for finance, which focuses on cost, revenue, and economic forecasts.
Using a uniform Business Analytics tool across these departments can lead to scenarios where the tool does not fully support the sophisticated data visualization required by marketing or the regulatory compliance needed by finance. This can result in suboptimal data insights and potentially misguide strategic decisions.
For example, a Business Analytics solution that excels in financial forecasting may not necessarily be the best tool for analyzing customer behavior or managing supply chain logistics. When organizations adopt a one-size-fits-all approach, they often miss out on features that are more suited to specific types of data analysis, leading to suboptimal outcomes.
Commonly Misunderstood and Misapplied Advanced Features
Feature Complexity and Interface Challenges
Advanced features like machine learning models or complex data integrations in Business Intelligence tools can intimidate users due to their perceived complexity. For example, the use of R or Python scripts within BI reporting tools to run predictive models requires a level of programming knowledge that not all business analysts possess.
Solution: Implement user-friendly interfaces that provide guided analytics and simplified access to complex functionalities. For instance, BI tools could incorporate natural language processing (NLP) to allow users to make complex queries without needing to write code. Additionally, providing access to pre-built models and wizards that guide the user through the analytics process can help demystify these features.
Technical Breakdown of Misunderstood Features
Predictive Analytics
Technical Barrier: The integration of predictive analytics often requires a deep understanding of statistical modeling and data science principles, which goes beyond traditional BI competencies.
Solution: Develop a suite of template-driven analytics models tailored for specific industries and departments. For instance, create retail-specific models that automatically adjust for seasonal trends and promotional impacts, or finance-specific models that are attuned to economic cycles.
Data Mining
Technical Barrier: Effective data mining requires not just an analysis across broad data sets but also the ability to clean and preprocess data effectively, which involves handling missing values, outlier detection, and ensuring data normalization.
Solution: Enhance BI reporting tools with automated data preprocessing modules that can detect and correct common data issues before the mining process begins. Integrate visual data mining tools that allow users to easily segment data and visualize patterns without requiring extensive statistical knowledge.
Real-Time Data Processing
Technical Barrier: Real-time data processing demands robust infrastructure and the capability to integrate streaming data effectively with batch-processing data systems.
Solution: Leverage cloud-based BI solutions that offer scalability to handle large streams of real-time data. Implement hybrid transactional/analytical processing (HTAP) capabilities to enable real-time analytics on transactional databases without the need for additional data movement.
Conclusion
As we have explored throughout this discussion, the perceived intelligence of your Business Analytics tool might not fully align with reality. From the challenges of maintaining data quality to the pitfalls of over-reliance on automation, it's clear that while these tools are powerful, they are not infallible. They require a keen understanding of their capabilities, careful integration into business processes, and ongoing management to truly deliver on their promises.
The key takeaway is not to dismiss your current analytics solutions but to enhance how you use them. It’s about recognizing their limitations and proactively filling the gaps—be it through better data practices, enhanced training, or more tailored solutions. This approach will not only improve your current analytics outcomes but also pave the way for more sophisticated use of these technologies.
For those looking to explore new analytics solutions or enhance their current tools, consider experimenting with advanced platforms that offer more customization and integration flexibility. For instance, Grow provides a dynamic Business Intelligence tool tailored to meet specific business needs, allowing for more precise and actionable insights.
With Grow, you can take advantage of a 14-day free trial to see how its capabilities align with your business goals. Check out Grow.com Reviews & Ratings 2024 on TrustRadius for unbiased user feedback and see how Grow stands out in the marketplace.
Remember, the goal of business analytics is not just to collect data but to drive meaningful business decisions. As you move forward, take a moment to reassess your tools, refine your strategies, and ensure that your business intelligence is as smart as you need it to be. Explore, experiment, and expand your analytics capabilities with Grow, and take your data-driven decision-making to the next level.